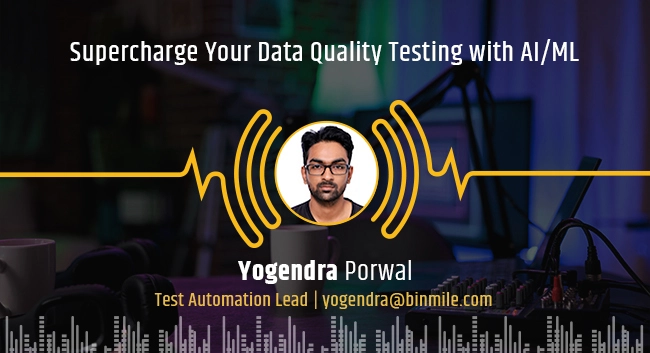
Podcast: Transforming ETL Testing with AI/ML
Welcome to our podcast page, where we delve into the transformative power of AI and Machine Learning in the realm of ETL testing and data quality. In our latest series, โTransforming ETL Testing with AI/ML,โ we explore how these cutting-edge technologies are revolutionizing the way we manage and maintain data integrity. This podcast is brought to you in collaboration with Binmile, a leader in digital engineering and quality assurance services.
What Youโll Discover in the Podcast:
- AI-Driven Innovation: Understand how AI and ML are enhancing traditional ETL processes, leading to more efficient and accurate data quality testing.
- Advanced Techniques: Discover the latest AI/ML techniques for automated anomaly detection, data profiling, and self-healing data pipelines.
- Expert Perspectives: Gain insights from industry experts on the practical applications and benefits of integrating AI/ML into your data testing strategies.
- Real-World Examples: Learn from real case studies on how AI/ML has improved data quality across various industries.
- Future Trends: Explore the future of data quality testing, including predictive maintenance and AI-powered data validation.
Podcast Highlights
Understanding Data Quality and ETL Testing
- Data as the New Gold: In today’s data-driven world, organizations rely heavily on high-quality data to make informed decisions. Whether it’s predicting stock market trends or enhancing customer experiences on e-commerce platforms, the accuracy and reliability of data are paramount.
- The Role of ETL Testing: ETL (Extract, Transform, Load) testing ensures that data moving through various stages of the process is accurate, consistent, and meets the necessary quality standards. This is critical for generating trustworthy insights from data.
Approach to Data Quality Testing
- Domain Understanding: Knowing the specific domain (e.g., financial, telecom, customer data) is crucial for tailoring the data quality approach. For instance, financial data demands high precision, while customer data may focus on diversity and lack of bias.
- End-Use Consideration: The purpose of the processed dataโbe it for recommendations, training LLMs, or generating financial insightsโdetermines the specific quality checks and validations required.
Challenges in ETL Testing
- Resource Constraints: QA teams often face tight deadlines and limited resources, particularly in ETL testing, where data complexity and lack of automation can pose significant hurdles.
- Data Complexity: Managing diverse data sources and evolving formats adds to the challenge, making it difficult to maintain consistent data quality.
- Size of Data: As data dimensions grow, manual profiling and validation become impractical, necessitating advanced tools like AI to ensure data quality.
AI/ML and Its Potential in ETL Testing
- Transforming ETL Testing: AI/ML is set to revolutionize how ETL testing is conducted. While it won’t replace traditional methods entirely, it can significantly enhance them by automating monotonous tasks and uncovering deeper insights.
- Automated Anomaly Detection: AI/ML can detect hidden patterns and real-time anomalies in data, which may go unnoticed in manual testing, thereby improving data reliability.
- Enhanced Data Profiling: AI/ML offers deeper insights into data, revealing complex relationships and generating intelligent quality rules that reduce manual effort.
Implementation and Impact of AI/ML in ETL Testing
- Real-World Application: AI/ML tools like Collibra and QuerySurge are already making strides in data validation and anomaly detection, leading to better data quality and governance.
- Data Profiling and Anomaly Detection: Case studies have shown how AI can enhance data profiling and anomaly detection, providing richer insights and better testing coverage.
Considerations for AI/ML Adoption
- Data Quality: The effectiveness of AI/ML models hinges on the quality of the training data. Ensuring data accuracy and completeness is vital for successful AI implementation.
- Infrastructure and Expertise: Implementing AI/ML requires significant computational resources and domain expertise, making it essential to plan for infrastructure and talent needs.
- Model Monitoring and Integration: Continuous monitoring and retraining of AI models are crucial for maintaining their effectiveness. Seamless integration with existing tools and workflows is also key to successful adoption.
The Future of Data Quality Testing with AI/ML
- Efficiency and Accuracy: AI/ML will drive efficiency by automating routine tasks and enhancing accuracy through advanced data analysis. This will allow testers to focus on more complex challenges, leading to higher quality outcomes.
- Proactive Data Quality Management: AI’s ability to predict potential issues before they occur will shift data quality management from a reactive to a proactive approach, ensuring data integrity from the outset.
Conclusion and Advice
- Exploring AI/ML: As AI/ML continues to evolve, it’s crucial for quality analysts to stay informed and explore these technologies. Understanding how they work and the challenges they bring will empower testers to harness their full potential in improving data quality.
This concept note outlines the key themes and insights from the upcoming podcast, providing a structured overview of how AI/ML is poised to transform ETL testing and data quality practices.